AirDNA Forecast Capabilities
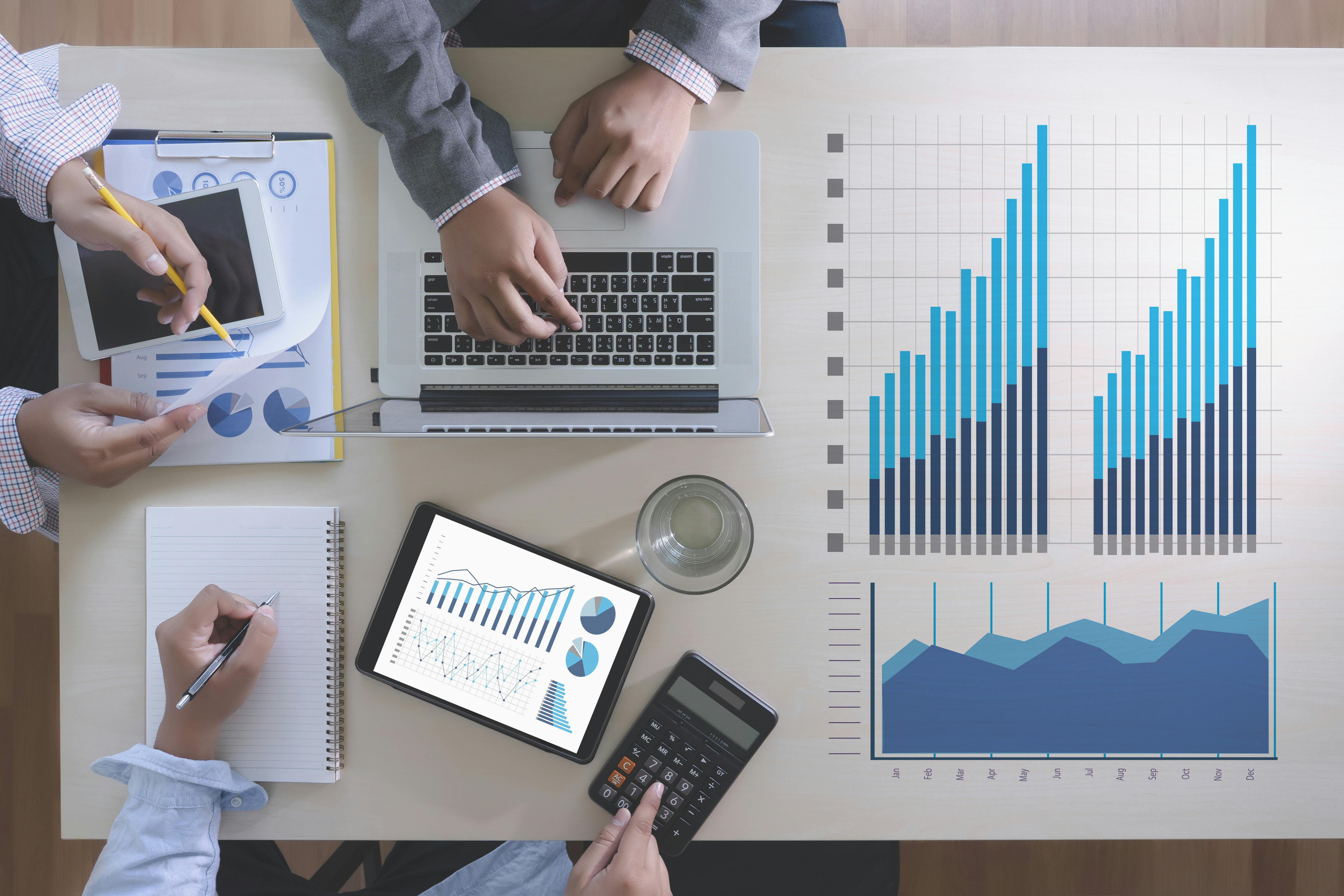
AirDNA has long published current and historical performance data for short-term rentals (STRs) globally from scraped data. The uses for this data range include benchmarking property performance, providing guidance when setting rates, and understanding past episodes of compression. In addition to these useful metrics for operators, the data can also be used to inform investment decisions. The data can point to which lucrative STR markets you should target with Airbnb efforts as well as which types of investment properties are most suitable for a given location.
In all of these use cases, but especially for investment activities, the underlying assumption is that present and past performance gives some indication about future performance. Now, AirDNA can provide users with an explicit forecast: a well-considered opinion of future performance that incorporates the effect of economic variables, future events, and historical seasonality. Additionally, AirDNA’s proprietary algorithms determine reservations for future dates, which speak to present attitudes toward future travel.
In its current form, the AirDNA forecast encompasses U.S. national-level performance, six U.S. location types, and the top 50 U.S. markets that AirDNA tracks most closely. We predict monthly variables, including demand, nights supplied, and revenue. In addition, the forecast measures occupancy, average daily rates (ADR), and revenue per available rental (RevPAR), as well as year-over-year (YOY) percentage changes.
We construct these forecasts by combining two methods for both short and long-run views on performance. For short-run forecasts, looking at bookings that have already been made for a future date (advance bookings) can make highly informative predictions. However, for longer horizons of four months or more, advance booking is not very informative. The lead times in the STR market, or how far in advance the guest reserves their Airbnb, are often quite short.
Instead, we make use of the robust, proprietary economic forecasts from Oxford Economics to create an econometric model that relates STR performance to several economic variables.
Short-Term Forecasting: the Booking Curve
One of the most reliable sources of information on future stays is the booking that has already happened for that future date. For any given date, the number of people that have made reservations increases as we approach the date. When we finally arrive at the date, 100% of the people that will reserve a property for that date have booked.
When you plot the proportion of people that have booked as you approach a date, a very consistent, predictable curve emerges under normal circumstances. We call this the booking curve.
Hospitality researchers have brought up the exponential function as a reliable function to fit booking curves. Therefore, you can make a forecast by using earlier portions of the booking curve to predict the later portions.
Examining Denver’s June booking curve, for example, we see that the booking curves lay on top of each other. There is a notable exception for the year 2020, when Covid-19 hit, and to a lesser extent in 2021.
In addition to the exponential function, there is a small dip in the last data point when you use monthly aggregates. People staying during the first half of the month have less time during the month to book as those staying in the latter half, after all!
In our forecasting, we observe that the shape of the curve varies seasonally and each market has its own distinct shape. Because of that variability, we estimate the booking curve separately for each market and for each month in that market.
The change in average lead time for bookings is the last factor to consider when using the booking curve to make predictions. The average lead in booking is affected by both the season and longer-term trends. These changes will shift the booking curve up or down depending on whether the lead time shortened or lengthened, respectively.
When you compare overall lead times between Atlanta and Denver by month, you start to see a strong seasonal variability in the case of Denver and remarkably short lead times year-round in Atlanta.
The useful forecast horizon also changes with lead times. Denver’s July booking curve provides useful predictions for three or four months out, whereas Atlanta’s curve only produces useful forecasts for perhaps two months into the future.
Medium-Term Forecasting: Econometric Model
For forecast horizons longer than three or four months, booking curves are insufficient. This is true even in markets with relatively long lead times—they just aren’t long enough to provide much information about events far in the future.
For medium-term forecasts, we switch to using longer-term economic variable forecasts from Oxford Economics and relate these to historical performance in the various STR markets.
Higher incomes and higher gross metro product indicate growth and future increases in demand. However, inflation can affect prices. Supply is strongly related to interest rates and their effect on typical payments for new mortgages. When mortgage payments are too high relative to typical income, we expect supply growth to slow.
Nationally, the ratio of STR revenue to typical mortgage payments peaked in July of 2021. Six months later, in January 2022, supply growth peaked. Since then, both variables have been steadily declining. The time between the observed ratio of STR revenue to mortgage payments, and the later change in supply, indicates that this ratio is a leading indicator and is well-suited to forecasts.
Economic conditions locally and nationwide can have effects on travel and demand for STRs. In addition, our model captures other important data features such as seasonality. We account for the interdependence of variables as well as variables changing over time in long-run growth trends in the market. This means that if ADR increases sharply in a market, modeled demand would respond by declining. The occupancy would therefore decrease, limiting future ADR growth, and so on.
Capturing True Market Dynamics: Repeat-Rent Index
Another feature that has proven useful in our forecasts of future market performance is the use of the AirDNA Repeat Rent Index (RRI). The RRI tracks the nightly rates of existing listings, as opposed to ADR which averages rates for both old and new listings. RRI essentially provides a measure of rates that removes the effect of changes to the mix of properties in a market.
For instance, in 2020 and 2021, ADR increased sharply while RRI rose much more gradually. Demand for short-term rentals and a willingness to pay for them did increase in this time period, but much of the increase in ADR can be attributed to a shift in guest preferences to larger properties. Travelers were eager to vacation in less densely populated areas in Airbnbs with more bedrooms to support families “quarantining together” in larger homes.
On the other hand, ADRs retreated in 2023 while RRI stayed flat. This indicates that, on average, existing properties didn’t lower their rates. Rather, new listings in 2023 had lower nightly rates than existing listings already in the market.
Both measures are useful because they can be used to describe different things. RRI tells us how much existing operators in the area are changing their prices, while ADR tells us how much guests are typically paying in the market. The forecasts include both measures.
Uses of the Forecast: Your Listing in Relation to the Market
Stakeholders can use a forecast in many different ways. One way, called the “share-down” method, is to assess the performance of a particular listing as a ratio to the market performance.
That is, if a market ADR is $100 and a listing has been trending at $120, we would say it has a 120% market penetration for ADR. Using this method, market changes can be applied to a listing or portfolio of listings. Even an entire submarket’s performance could be predicted with the share-down method!
Furthermore, STR owners can make assumptions based on the trend of the penetration. If a property’s ADR is higher than the market ADR, recently installed new amenities may have driven it up. Or, new competition close by might drive it down, for instance.
For the rental manager that would like to frame owner expectations, this setup can provide satisfactory, evidence-based results. Plus, it uncovers vital information specific to their rental.
Destination marketing organizations (DMOs) who want to assess the anticipated economic impacts from tourism in their market over the next year can easily use revenue forecasts. These forecasts may also be useful when considering the next year’s budget, as occupancy taxes are typically a percentage of total rental revenue.
Investors can use forecasts to gauge what prices consumers will be willing to pay in future periods on average, and which markets are expected to grow the most over the next year or two.
The new forecasting capabilities at AirDNA can be used by anyone in the industry seeking a forward-looking, objective picture of the U.S. STR market. Our forecasts are powered by the industry’s best data and most advanced modeling technology.